In his PhD thesis, Pablo Durán has developed a novel coupling framework utilizing the Weather Research Forecasting (WRF) model with WindSim’s computational fluid dynamic (CFD) model for wind resource assessments.
The global demand for renewable energy has risen steadily in the past decade. The most important factors for the profitability of a wind energy project is the total amount of energy produced. This is estimated through a process called wind resource assessment.
Modelling wind flows
“The main goal of wind resource assessment is to predict the windiest locations within a given area. Due to the cost, it is only possible to concurrently measure the wind speed in a limited number of locations,” Durán explains.
Therefore, a method is required to extrapolate few measurements to other locations of interest. For this purpose, the wind industry typically uses the so-called numerical wind flow models.
“These models are designed to predict the spatial variation of the wind by modelling the physical behavior of the wind flow.”
How to deal with complex weather?
One of the most advanced wind flow models utilized in the wind energy industry for wind resource assessment are the steady-state computational fluid dynamic (CFD) models.
“These models have proven to be successful in modelling the wind flow in complex terrain,” Durán says.
“However, there are some limitations in their applicability at sites with complex weather patterns.”
Coupling models
In his PhD thesis, Durán has addressed these limitations by coupling WindSim’s CFD model with the WRF mesoscale model. Mesoscale models are widely used for weather forecast and can reproduce the complex weather phenomena that a CFD model lacks.
In his study, the framework to couple both models consists in utilizing the mesoscale simulation results to compute the boundary conditions of the CFD model.
“I have developed two meso-microscale coupling approaches,” he says.
Average values
Durán’s first approach consisted in utilizing the average values of the mesoscale fields by wind directional sector.
“This approach improves the wind estimations in complex terrain and in areas that are located at the wake of the terrain features of a site,” Durán says.
“Nevertheless, the approach presents important limitations in sites where the wind blows from few wind directions”.
Extracting weather patterns using machine learning
The second approach addresses this limitation by extracting weather patterns from the mesoscale simulations by means of a fully automated clustering methodology. “This classification technique is capable of extracting the predominant weather patterns and organizing them in a meaningful way”, Durán says.
He applied this approach in a desertic site with challenging weather conditions and extreme diurnal cycles. “By downscaling the extracted patterns of this site, the overall modelling error is reduced compared with the mesoscale model”.
“In addition to wind resource assessment, this methodology has a lot of potential for wind turbine wake studies as well as for forecasting solutions that utilize CFD models,” he concludes.
Durán defends his thesis “A novel framework for coupling mesoscale and steady-state CFD models for wind resource assessment” Tuesday the 12th of May, 2020.
Fakta
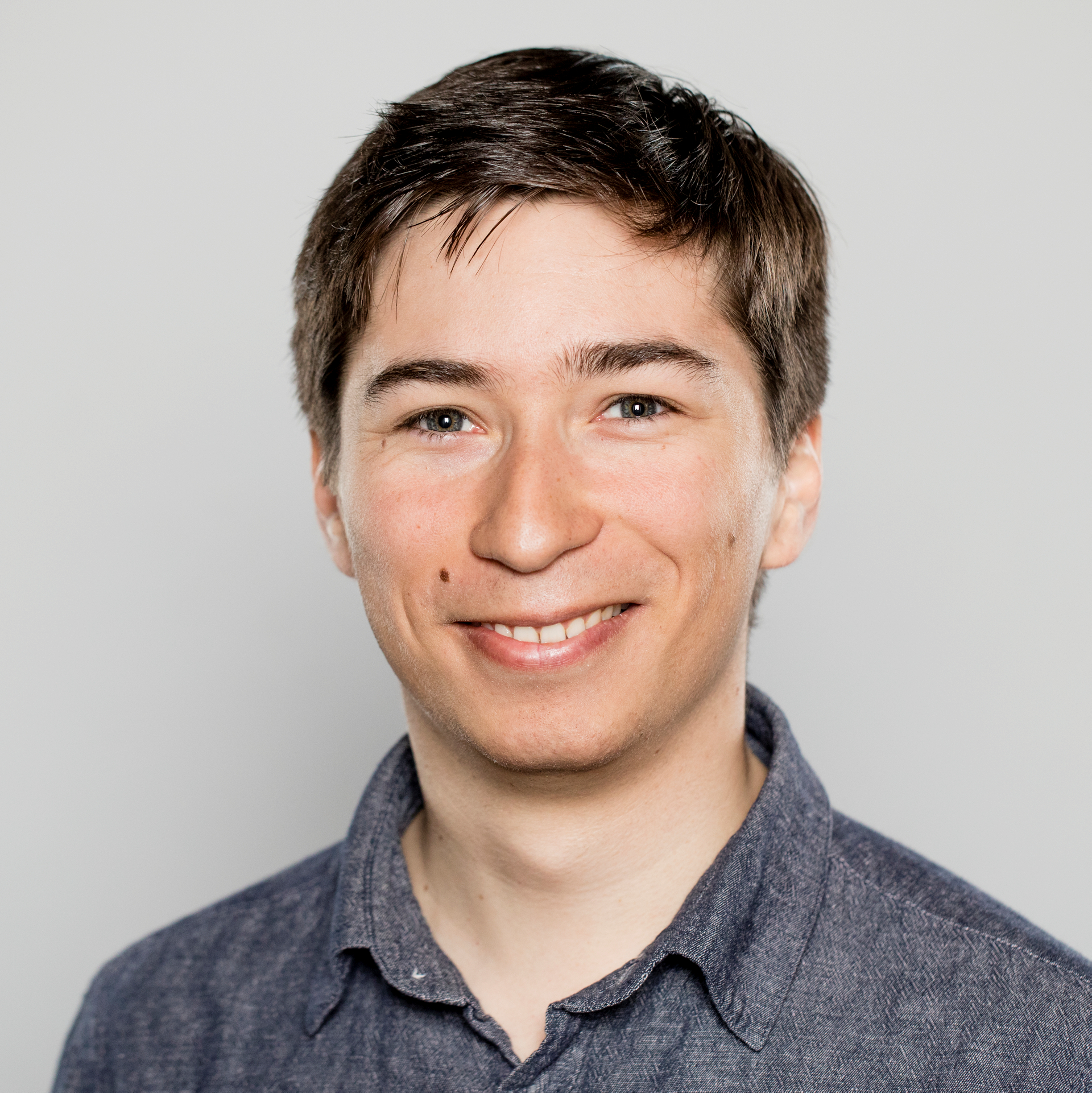