What does tradition and technology bring to the future of forestry?
The morning was beautiful. The sun had already risen above the horizon, yet shadows of the night still lingered in the forest opening along the road. The mountains stood in the distance, their twilight-blue silhouettes silently and steadily marking the landscape. The road curved like a river running through the ancient forest. There were no others — only these two cars on the road. They were alone, their electric engines echoing in the forest, their headlights piercing the morning mist.
They say that the mist is the dance of forest faries, appearing when the day trades places with the night, and the night with the day. A beautiful allegory, one could say. The two drivers knew of the ancient Norse mythology, and they especially enjoyed the morning drive, feeling a deep sense of belonging to their ancestry.
They were heading to the Våler experimental forest area, where Kåre was already waiting for them. The drivers were representatives from the two largest forest companies in Norway. They were important people — real big cheeses, as an American would say. They were used to making calculated, precise decisions, following the cold logic of profit. These days, however, it was becoming harder and harder to turn a profit. New legislation on timber quality and certification, forest reservations, carbon storage initiatives — all of it needed to be taken into account, woven into calculations, and factored into solutions for maintaining profitability. Not an easy task, one could say. Not an easy one at all.
Waiting for them was Kåre — a man of incredible skill, honed through decades of experience. Starting as a young boy who grew up on a farm by the forest, he elevated ocular assessment to an art of near precision and accuracy. From an early age, when he first learned about the relascope — invented by Walter Bitterlich, then at the forefront of forest inventory — he became fascinated by the idea of developing his body as an instrument for forest assessment. In other words, he never carried any tools with him, and yet he could assess tree height, basal area, diameter, and even the site index with utmost accuracy — often even surpassing modern instruments.
How did he do it, one might ask?
Kåre had turned his thumb into his own relascope. Every morning, he measured the width of his thumb and the length of his arm, adjusted his calculations, and with that knowledge, proceeded with his assessments. Over decades of calculations and measurements, he had trained his intuition to determine the site index with just three gentle jumps — not two, not four, not even two and a half, but exactly three. Gentle ones. Every time, it worked like a charm. He had once considered adopting a method for height measurement that he learned during a short visit to Russia, but eventually gave it up, realizing the true meaning behind it. Even now, he would blush and feel a wave of confusion when he remembered learning it — and doing it.
"Merkelig land [Strange country]", he mumbled, blushing again.
It was a misty morning. In the small valley of the Våler forest, the fairies swirled in a wild, silvery dance, as if spun by unseen winds. Kåre shivered slightly. He had already remeasured his thumb and arm on site, adjusting for the cold and dampness in the air. He was early. He would have to wait.
This meeting was his chance to introduce his skills to the top forest companies in Norway — companies known for adopting the latest technologies in their pursuit of enduring profit. These technologies, born from the symbiosis of top researchers and industry, were part of an initiative called SmartForest, standing at the frontier of technological development based on data collected by drones, robots, and processed by AI.
And yet, here he was, trading in the skills embodied in his own body — a living instrument — in contrast to machines. He was nervous, but also excited.
While waiting, Kåre let his mind drift back to the time when he was training his living instrument in the tropical forests of Tanzania. It was a time of incredible self-growth and challenge. The forest there was not the one he had grown up in and loved. It was different — but still a living organism, with mists and trees stretching beyond the clouds.
There, he learned that his calculations needed to be adjusted rather thoroughly. Due to the rapid changes in humidity and temperature during the day — and depending on the landscape he was in — he had to measure his thumb’s width and his arm’s length several times a day. So, after all, he had to carry some instruments with him. This slight deviation from his motto unsettled him at first, but he reassured himself: the instruments were not used directly for assessments and thus might not be counted. It was a time of great learning and adventure for him...
Kåre looked around and felt a wave of love for his land. It was misty and cold — but it was his home.
The cars approached. Two Teslas parked next to his silver Saab. Two men stepped out of their cars and caught sight of the Saab.
"Hva i all verden [What in the world]?" said the first man, raising an eyebrow.
"Han må være svensk [He must be Swedish]," he added, shaking his head slightly.
"Trodde Saab var utdødd [I thought Saab was extinct]," muttered the second man with a crooked smile.
Kåre greeted them. The introductions were made.
He had selected a few spots — fewer than 40, but more than 30 — throughout the experimental forest. There, he established his relascope plots, conducted his measurements, performed his calculations, and built his model to predict the growing stock volume for the 900-hectare study area.
His model was based on ocular assessment and had a scientific name: the Ocular-Assessment-Based Model, or OA-based model — also known as Kåre’s model. This particular one was the OA-based model for Våler, April 2025.
Throughout the years, Kåre had built such models for the experimental area in Tanzania, covering 30 square kilometres over several consecutive growing seasons. He had developed models for different parts of Norway as well — from the mountains in the north to the valleys in the south — as well as in Sweden and the northern part of Russia.
Typically, he would choose a few plots, purposefully selected based on his inner compass — plots he sensed would be truly representative of the area of interest. This inner compass had been trained over decades of experience and relentless practice. Of course, Kåre was aware of the rather small sampling fraction: the area covered by his plots was less than 0.05% of the total. Yet his skill — tested and honed across countless forest types and conditions — enabled him to develop OA-based models of the highest precision. Precision that no intelligence, natural or artificial, could surpass. Zero variance.
The final result of Kåre’s OA-based Våler, April 2025 model was elegantly simple:
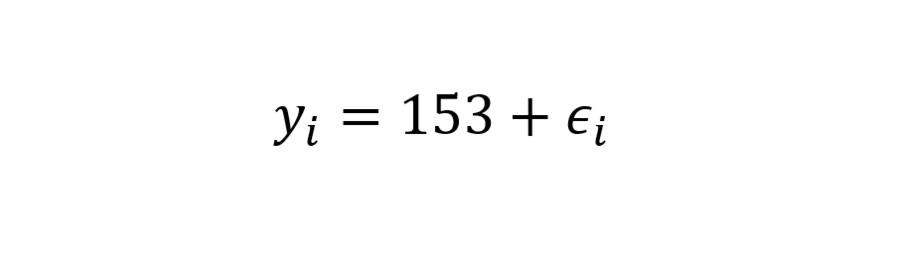
And the prediction of the area of interest population mean:
![y ̅ ̂=153 [m^3/ha]](https://main-bvxea6i-kdsvgmpf4iwws.eu-5.platformsh.site/sites/default/files/styles/original_ratio/public/2025-05/svetlanalikning2.png?itok=U0xoA7sR)
With the variance of prediction equal to zero, i.e.:
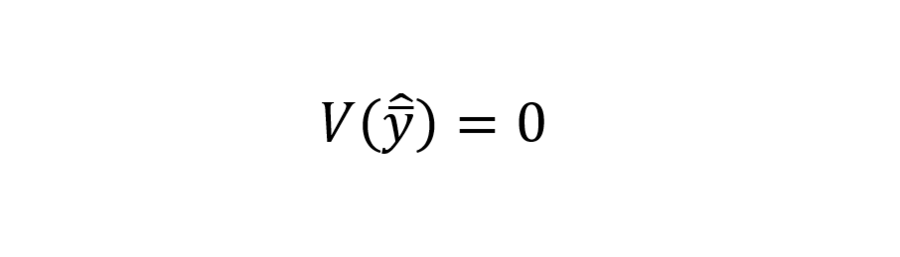
The two businessmen were amazed. Altogether, the entire process had taken them no more than nine hours — including walking from plot to plot and taking a short break for a modest sandwich wrapped in paper, accompanied by an apple.
And yet, Kåre’s estimate was precisely the same as the one they had obtained earlier by flying drones equipped with a multispectral laser scanning system.
The drones had collected terabytes of data, which were then processed by AI-based algorithms. The entire process — establishing field plots (maintaining a 0.15% sampling fraction), flying the drones, and processing the data — had taken more than two weeks. It had consumed kilowatts of energy — and in this era of energy crisis, that was a costly part: charging the drones, which drained power like thirsty camels drinking water in a desert, and charging the high-performance computers running the AI algorithms. There were other costs as well: paying the field crew and their high-tech instruments, and, of course, paying the operators who flew the drones and managed the AI systems.
After all this, they now stood watching Kåre — who had conducted his assessments and calculations in just under nine hours — arrive at the same result, with zero variance.
Of course, the variance from their drone-based method (calculated using some ad-hoc approaches, since formal methods for variance estimation in AI-based forest predictions do not yet exist) was also very small — close to zero, thanks to the intensive coverage provided by remotely sensed data collected by drones. But the cost of achieving it had been enormous.
They were stunned. Even "stunned" would have been an understatement. They were deeply shocked, immediately calculating — in their minds — the potential value of Kåre and his model for their companies.
It was Kåre’s triumph. And he was humbled.
The men returned to their cars. It had been a day where faith in innovation met the quiet authority of tradition.
Before leaving, the two businessmen shook Kåre’s hand.
"Tusen takk for i dag, Kåre [Thank you for today, Kåre]," said the first one.
"Kjør forsiktig [Drive safe]," said the second.
They were men of few words, but enormous action. Their minds were already strategizing how what they had just witnessed could be brought back home and woven into their operations alongside modern technologies.
After all, inclusiveness and openness were their motto.
Epilogue
In this context, how would an AI-based model differ from Kåre’s OA-based model, and would it differ in the statistical sense?
Both the AI-based and OA-based models are ultimately rooted in field plot measurements, serving as the anchor for their predictions. The AI-based model relies on vast amounts of remotely sensed data and algorithmic processing to refine its outputs, offering precision through computational power.
Kåre’s OA-based model, by contrast, achieves precision through human intuition, decades of embodied experience, and a direct, minimalistic engagement with nature. One multiplies data to approximate understanding; the other distils years of living knowledge into simple, decisive assessments.
Both approaches result in a very narrow confidence interval; however, neither (at least currently) has a statistical method for estimating the prediction interval, which is based on the mean squared error, as opposed to the confidence interval, which is based on the variance.
In other words, the assessed uncertainty relates to the model’s expectation rather than the actual true value, which can be problematic, since both models may be severely biased — and such bias may be neither detectable nor estimable. Thus, while their methods differ, the ultimate nature of their predictions — and their uncertainties — remains fundamentally similar.
Disclaimer and author's note
This story is a reflection on current technological developments in forestry. It is meant to raise awareness about the direction the field is taking, without minimizing the importance of technological advancements.
It is not intended as a criticism nor as sarcasm toward modern methods, nor toward the mastery of field skills and human intuition. Rather, it is a tribute to the complementary strengths that both tradition and technology bring to the future of forestry.
This text was written by the author with assistance from ChatGPT-4.0, which was used for checking English and Norwegian grammar and refining language flow.
The accompanying figure was generated by ChatGPT-4.0 with the assistance of the author.